Machine Learning and Infectious Disease
Dr. Adriana Tomic is a Marie Curie Fellow at the Oxford Vaccine Group, Department of Pediatrics. She is a systems immunologist applying machine learning to understand how vaccines work, specifically focused on human immunology and data-driven research. Co-developer of SIMON, an open source platform for the application of machine learning to biological and clinical data.
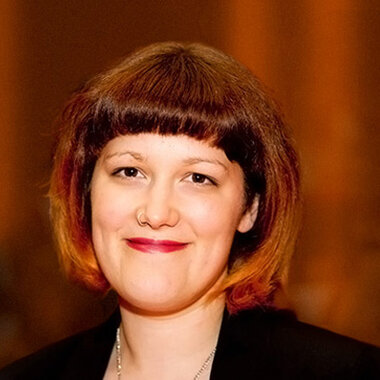
In one of the 20 most-read papers of 2019, Stanford University and University of Oxford researchers developed an automated machine learning system, Sequential Iterative Modeling “OverNight” (SIMON), that compares high-dimensional datasets from heterogeneous clinical studies focusing on vaccine response (Figure 1). The aim of the project is to build more accurate predictive models and accelerate analysis and discovery. “SIMON is a highly useful approach for data-driven hypothesis generation from disparate clinical datasets,” says Adriana Tomic, PhD, lead author on the paper.
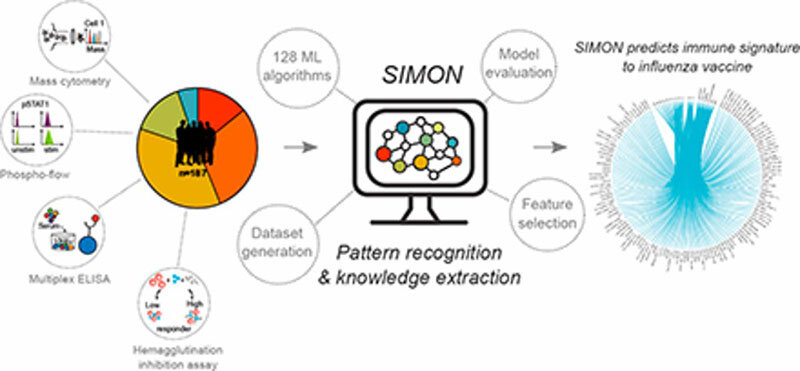
Blending machine learning with systems immunology
Taking a systems immunology approach to evaluate changes in immune cells and molecules during an infection or treatment can accelerate the understanding of an effective immune response. Integration of machine learning algorithms into systems immunology can further facilitate the discovery of unseen patterns in relevant immune cell subsets and genes. However, current approaches are challenged by heterogeneous or incomplete datasets. To overcome these obstacles and tailor machine learning to adapt to the variability of multiple clinical studies, Tomic and team developed SIMON open source software for the application of machine learning to high-dimensional clinical datasets. Put more simply, SIMON can predict if an individual will respond appropriately to a vaccine based on a set of immune system parameters.
SIMON was developed to improve computational analysis of influenza vaccination responses in humans, a task that is typically unreliable due to smaller sample sizes and single-target studies. Instead, SIMON analyzes multiple parameters from numerous individuals across several studies to accurately capture biological variability and to increase statistical significance. The inclusion of machine learning helps minimize sample loss and identify algorithms that fit any given data distribution, maximizing predictive accuracy and other performance measurements.
Initially, SIMON analyzed data from the Stanford Human Immune Monitoring Center (HIMC) in a novel project, FluPRINT. Five separate clinical studies of seasonal influenza vaccination were included, with various platforms and expanding parameters, including single-cell analysis at the gene and protein levels using mass cytometry to capture both immune system and individual variation. “By using SIMON, we identified subsets of immune cells not previously described to provide protection against the virus. These results are important for the development of the next generation of vaccines and have the capacity to fundamentally change vaccinology by application of machine learning to speed up discovery,” explains Tomic.
The data generated from this study was collected in an open-access FluPRINT database, meant to enable large-scale studies exploring the cellular and molecular basis of successful antibody responses to influenza vaccines. The resource can be used to uncover new markers and mechanisms that are important for influenza vaccine immunogenicity.
The move to any infectious disease
The SIMON systems immunology approach used in the FluPRINT project to predict flu vaccine response could also become crucial for understanding other infectious diseases, such as SARS-CoV-2 (Figure 2), and developing a vaccine to stop the COVID-19 pandemic. “Generating a similar dataset from ongoing clinical trials on SARS-CoV-2 will be critical for understanding this virus and generating an efficient vaccine,” says Tomic.
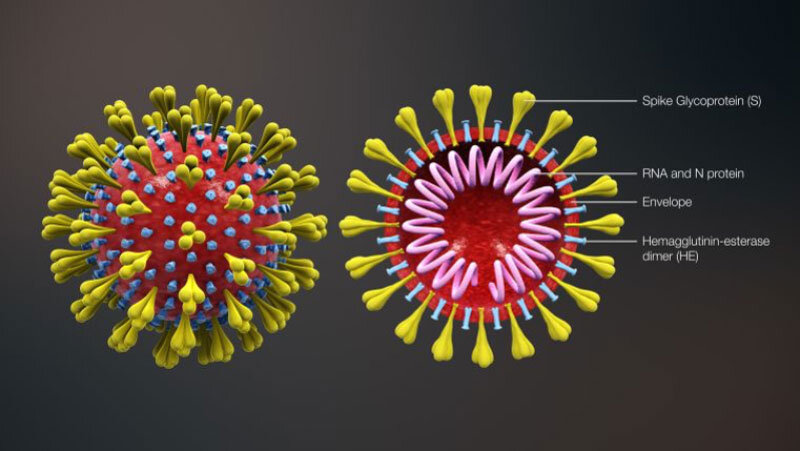
Evaluating interindividual variation in response to an infection or a vaccine aligns with clinical presentation of COVID-19, where such a wide and unpredictable range of immune responses is observed from patient to patient. SIMON could assist in determining which patients might progress to critical stages of the disease based on their immune profiles and which would respond to certain treatments. To this end, Tomic is part of a University of Oxford team that has now identified a SARS-CoV-2 vaccine candidate and is working toward the first clinical testing phase.
The FluPRINT project is described in detail at fluprint.com and SIMON is available for download at genular.org.
Read the official release about the new vaccine candidate against COVID-19: ovg.ox.ac.uk/news/covid-19-vaccine-development
For Research Use Only. Not for use in diagnostic procedures. Patent and License Information: www.standardbio.com/legal/notices. Trademarks: www.standardbio.com/legal/trademarks. Any other trademarks are the sole property of their respective owners. ©2025 Standard BioTools Inc. All rights reserved.